Reproducibility is an important topic for Machine Learning research and we had as invited speaker Koustuv Sinha in our colloquium (14th of October). He is one of the co-organizers of Machine Learning Reproducibility Challenges on (basically all the) major ML conferences over the last five years. He provided a very nice overview on the need of reproducibility and in particular we discussed best practices for projects.
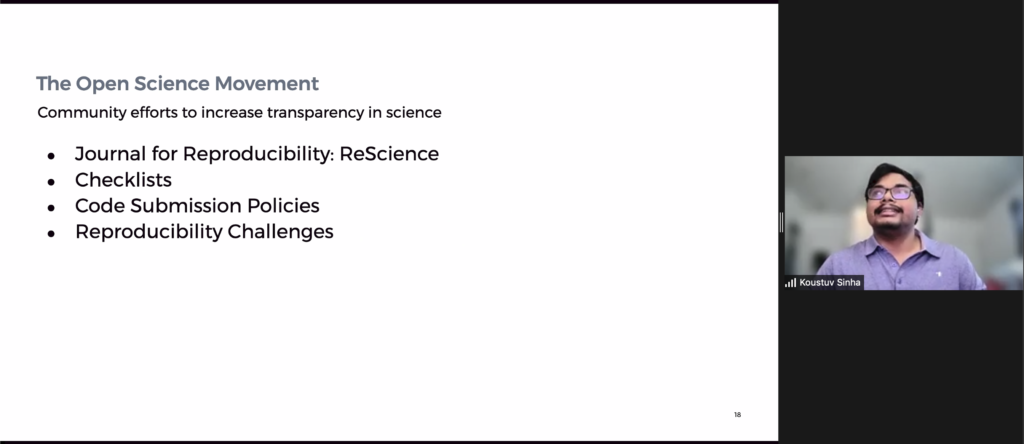
Title: Hands on Reproducibility – A primer on reproducible research in ML
Abstract: The incredible progress in the field of Machine Learning over the last five years have pushed the state-of-the-art in many applications across different modalities. However, translation of these findings to real world problems has been slow – primarily due to issues in reproducibility. Reproducibility, which is obtaining similar results as presented in a paper or talk, using the same code and data (when available), is a necessary step to verify the reliability of research findings. Reproducibility is also an important step to promote open and accessible research, thereby allowing the scientific community to quickly integrate new findings and convert ideas to practice. Reproducibility also promotes the use of robust experimental workflows, which potentially reduce unintentional errors. In this talk, I will first present some statistics on the need for reproducibility in machine learning research, and then cover the recent approaches taken by the community to promote reproducible science. Finally, I will talk in-depth about the experimental workflows that you can integrate with your research, to ensure and promote reproducible science.