Goal
Many application areas require resource-efficient computations, for example, computer vision tasks in production. Artificial Intelligence solutions that allow to adapt online to changing conditions in such tasks are promising, but often involve large models and costly computations. This project aims at exploring in how far biological-inspired spiking neural network architectures can be employed in hardware which, on the one hand, allows for efficient computation, and, on the other hand, facilitates online adaption and learning.
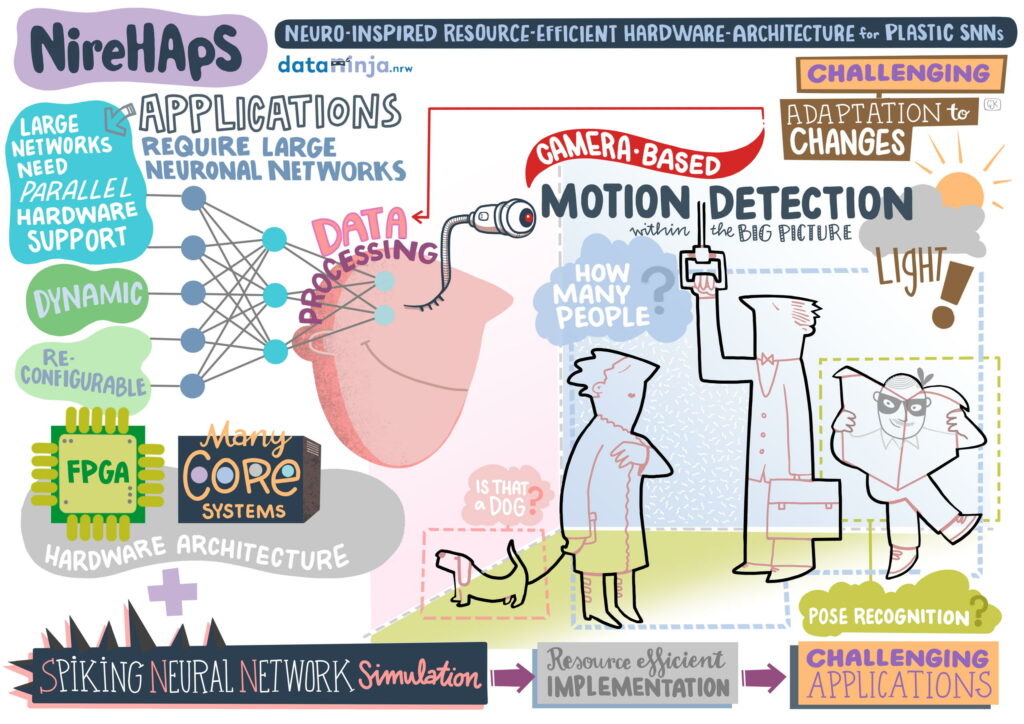
Project Overview
Implementing online learning methods into resource efficient hardware will allow to embed such methods directly on sensor hardware, reducing high-bandwidth communications and allow for faster processing. The project will use current embedded (and many core) hardware architectures for testing online learning methods directly in hardware and applied in spiking neural networks. As one example, these systems will be employed for ultra-high-speed computer vision and event detection.
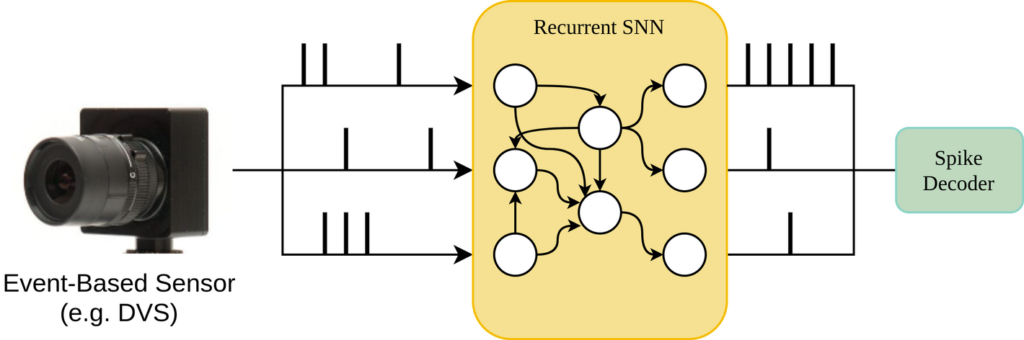
The project will explore the design-space of possible embedded hardware architectures and analyze resource-efficient implementations of biologically-inspired spiking neural networks. The different architectures will be applied in Artificial Intelligence tasks as, for example, in online learning in computer vision. First, with respect to hardware architectures this will cover reconfigurable hardware platforms as FPGA and application specific many-core systems. Secondly, different neuron and synapse models – as well as configurations of these model – will be evaluated, for example variations of spike-timing dependent plasticity. The hardware platforms will finally be coupled with event-based sensors (e.g. DVS cameras) to evaluate the solutions on the basis of practical application scenarios.
1–4
Target platforms are FPGAs and multi-core processor architectures. UBI uses high-level synthesis (HLS) and parameterizable VHDL and Verilog code for mapping on FPGAs. For online learning, the weights of neurons and synapses can be adjusted during runtime, or changes to the network structure can be achieved by replacing the bitstream (reconfiguration or (partial) dynamic reconfiguration). Utilizing the synaptic delay enables larger networks to be mapped without restricting the maximum clock frequency. The specified synapse delay between distant neurons can be implemented by memory elements (several clock cycles). The event-driven processing supports hierarchical clock gating, and sensible network segmentation (grouping of groups of neurons that are often firing simultaneously in clock regions) offers potential for optimizing energy efficiency.
The FHBI examines the suitability of the multi-core and RISC-V-based architectures as specialized processor architectures. For these, the integration of special hardware extensions (e.g., instruction set extensions, VLIW, vector extension) is examined. Many-core architectures are typically based on a hierarchical communication infrastructure with shared resources (e.g., shared bus, shared memory) and a global network-on-chip (NoC). The analysis of the hardware implementation of the SNN provides information about which operations (properties of the neuron model, complexity of the learning rules) have a significant influence on resource efficiency. The application of the above-mentioned learning strategies for online adaptation is examined for both target architectures. Also, the target architectures will be coupled with event-based sensors for evaluating the solutions of ultra-high-speed computer vision and event detection tasks.
Preliminary Results:
As for the first assessment, we have done the literature research on spiking neural networks and simulated the SNNs using the brian2 simulator to understand the behavior of the neurons and neuronal models. For getting hands-on with practical applications, we realized a Binary Neuronal Associative Memory (BiNAM) using SNNs [1]. We investigated how the storage efficiency behaves with varying samples and other essential model parameters like threshold and weights. The critical model parameters are determined by performing multiple experiments.
On the other hand, to perform in silico analysis, in order to gain accurate parametric values and observe the complete behavior of an SNN model, a different set of input conditions, i.e., what values are required, which component values are dependent on each other, must be defined in each event. Even though the group of neurons is moderate-sized, the combinations of these component inputs into the model may require very precious values to be defined and simulated. However, a significant amount of dedicated simulators have been developed to analyze and visualize the SNNs behavior. Such simulators are providing the users to acquire precise simulations in a relatively short period of time. Nevertheless, there are many challenges and computational issues related to SNN. In some cases, it requires the use of accurate biological representations of the neurons. Alternately, a real-time capability with the model provides a more appropriate way to make direct changes at any instant of time in the simulation to observe the model behavior.
With this motivation behind and the existing challenges in understanding and leveraging the promising features of SNNs, we present the first version of our novel spiking neural network user-friendly software tool named RAVSim (Run-time Analysis and Visualization Simulator), which provides a runtime environment to analyze and simulate the SNNs model [2]. It is an interactive and intuitive tool designed to help in knowing considerable parameters involved in the working of the neurons, their dependency on each other, determining the essential parametric values, and the communication between the neurons for replicating the way the human brain works. Moreover, the proposed SNNs model analysis and simulation algorithm used in RAVSim takes significantly less time in order to estimate and visualize the behavior of the parametric values during a runtime environment.
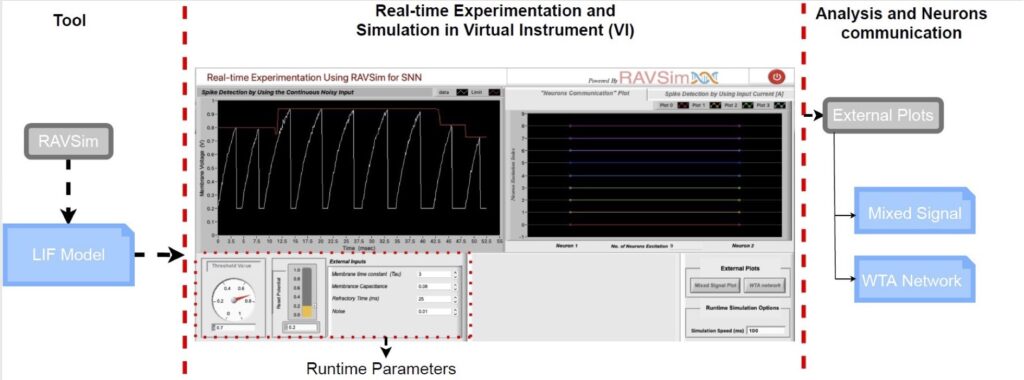
RAVSim Availability:
The RAVSim (v1.0) is an open-source simulator
- it is published on LabVIEW’s official website and available publicly at
https://www.ni.com/de-de/support/downloads/tools-network/download.run-time-analysis-and-visualization-simulator–ravsim-.html#443936 - video demonstration can be accessed on our Youtube channel: https://www.youtube.com/watch?v=Ozv0MXXj89Y
- user manual is available on our GitHub Repository: https://github.com/Rao-Sanaullah/RAVSim/tree/main/User-Manual
Cooperation
Project Publications
- Shamini Koravuna and Ulrich Rückert „Spiking Binary Associative Memory for Implementing on Neuromorphic Hardware. “ In KI 2021 44th German Conference on Artificial Intelligence. Workshop on Trustworthy AI in the Wild (Sept. 27, 2021). 2021. E-print: https://dataninja.nrw/wpcontent/uploads/2021/09/3_Koravuna_Spiking_Abstract.pdf
- Sanaullah, Koravuna, S., Rückert, U., Jungeblut, T. (2022). SNNs Model Analyzing and Visualizing Experimentation Using RAVSim. “In Engineering Applications of Neural Networks. EANN 2022. Communications in Computer and Information Science, vol 1600. Springer, Cham. https://doi.org/10.1007/978-3-031-08223-8_4”
References
- 1.S. Lutkemeier, T. Jungeblut, H. K. O. Berge, S. Aunet, M. Porrmann, U. Ruckert. A 65 nm 32 b Subthreshold Processor With 9T Multi-Vt SRAM and Adaptive Supply Voltage Control. IEEE Journal of Solid-State Circuits. 2013;48:8-19. doi:10.1109/JSSC.2012.2220671
- 2.Ostrau C, Klarhorst C, Thies M, Rückert U. Benchmarking and Characterization of event-based Neuromorphic Hardware. In: ; 2019. https://pub.uni-bielefeld.de/record/2935328
- 3.J. Ax, N. Kucza, M. Vohrmann, T. Jungeblut, M. Porrmann, U. Rückert. Comparing Synchronous, Mesochronous and Asynchronous NoCs for GALS Based MPSoCs. In: 2017 IEEE 11th International Symposium on Embedded Multicore/Many-Core Systems-on-Chip (MCSoC). ; 2017:45-51. doi:10.1109/MCSoC.2017.19
- 4.J. Ax, G. Sievers, J. Daberkow, et al. CoreVA-MPSoC: A Many-Core Architecture with Tightly Coupled Shared and Local Data Memories. IEEE Transactions on Parallel and Distributed Systems. 2018;29:1030-1043. doi:10.1109/TPDS.2017.2785799