Goal
Process mining is an interdisciplinary field that aims to bridge the gap between data science and process science. Process discovery, conformance checking and process improvement are the three main types of process mining. In Process Mining we use knowledge extracted from event logs to uncover, monitor, and improve real business processes, allowing us to understand what is really going on in a business process rather than what we assume is going on. Event data is used to define and construct symbolic process models in various fields, including logistics, finance, hospital treatment, and industrial production. Process models may be learnt in this way, and they give a formal and interpretable description of the underlying events. In order to verify an existing model or uncover problematic sections of a model, process mining tools are frequently employed retroactively. The ML4ProM project intends to supplement such retrospective analysis with predictive Machine Learning technologies in order to comprehend the processes better and provide predictions to enrich the information provided by the process model. Furthermore, the project would also produce alternative solutions by discovering deviations from the model as early as possible. Currently our goal in the Tandem project is defined to connect these two research fields, focusing on benefiting from the machine learning techniques to improve process mining tasks.
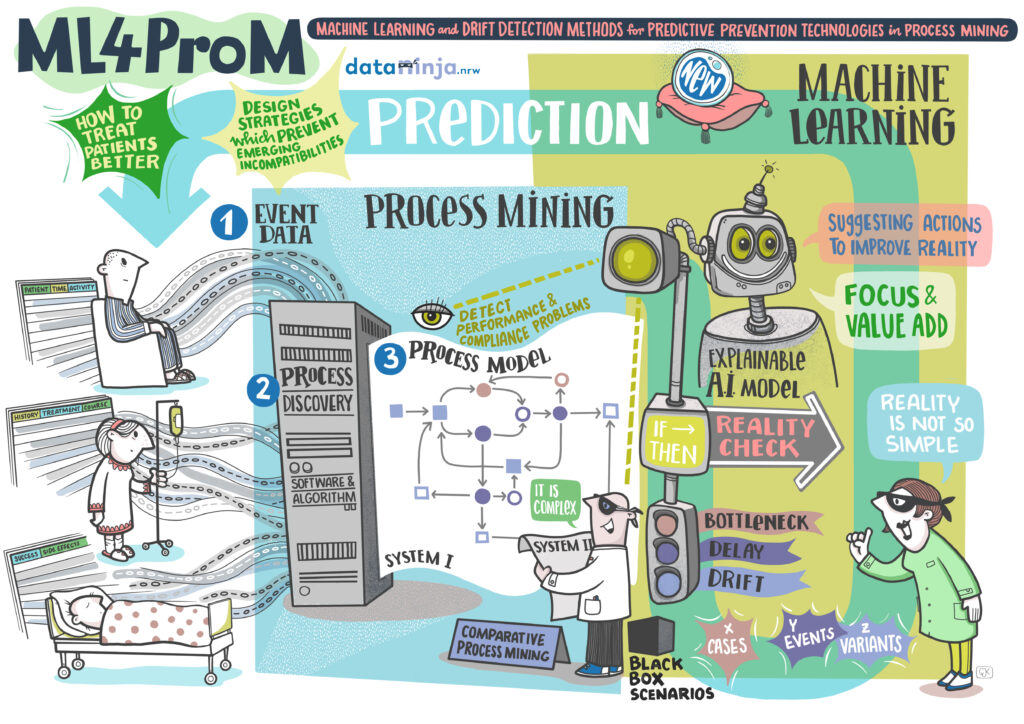
Process models are simple to understand and may be used to express predictions and actions available in the historical event data. But it fails to make predictions regarding the future events, processes, bottlenecks. In other words, the majority of process enhancement works focus on post-mortem analysis; that is, conformance approaches are reactive in the sense that they discover a violation after it has occured. Predictive monitoring approaches, on the other hand, are proactive in that they provide predictions before a violation occurs, therefore increasing the process performance and reducing risks. Hence, these kind of approaches are known as ‘forward looking forms of process mining’ which has predictive capabilities with the help of AI models being used. Recurrent Neural Networks(RNNs) are the main AI models used in predictive monitoring which is able to process sequential event data. One of the main goals of the project is to build such AI models leveraging the state-of-the-art architectures to provide predictions. Another task is to learn drifts of essential sections of (sub-)processes in the process model to prevent emerging incompatibilities. In addition, it’s critical to retain explainability in the expansion of learnt components, such as employing relevance learning to identify relevant aspects in connection to a process.
Project Overview
Machine Learning
Every human being in the planet is unique, and how we sense and act may differ from one another. Some people like to drink the tea with milk, some not. Some people like to work in the mornings, some prefer to work in the night. And when it comes to work, regardless of which industry, company, or department we work in, we do not always follow a prescribed path, policy, or work instruction. We have our own ways to do things, so to say, and this makes managing processes really challenging and unpredictable. Process Mining sheds a light on processes and generates a symbolic representation of them, which helps from the managing perspective. But it lacks the ability to ‘look forward’, in other words, it can not predict the future events/actions. This is where Machine Learning(ML) comes into play with its predictive capabilities.
The use of Artificial Intelligence (AI) in process mining leads to a new type of research, that is: ‘forward looking forms of process mining’, which has predictive capabilities that improve the process performance and reduce risks. But such AI models are usually black-box, meaning that models may not be interpretable. However, current advances on explainable AI (XAI) continue to shed a light on the model’s decisions. By using XAI methods as concerns the explanations of the model’s predictions we achieve interpretability as well as the predictive power.
Process Mining
Process discovery is a type of process mining that aims to discover a process model from an event log. Many discovery techniques are introduced to discover a comprehensive process model from an event log [1]. Process models are represented using different formalizations such as directly follows graphs, BPMN models, and Petri nets [2]. Several quality dimensions are proposed to check the conformance of a discovered model, such as fitness, precision, generalization, and simplicity [3]. Although these measurements provide insights into the quality of discovered models, deciding on an appropriate model depends on applications and expectations.
The selected research topic “Discovering Process Models That Support Desired Behavior and Avoid Undesired Behavior” is relatively new in the process mining field. The main focus of the current research project is on the development of an algorithm that has a desirable event log and an undesirable event log as inputs and discovers a Petri net model that supports desirable behavior and avoids undesirable behavior of the process. The problem was investigated from different aspects. Although there are some initial efforts to consider negative examples in process mining literature, still there is no comprehensive framework to do so.
Preliminary Results
As the first step, we designed some evaluation metrics to measure the improvements. We developed our algorithm based on Inductive Miner as a well-known process discovery algorithm. Our algorithm is recursive and each recursion searches for a cut, that has the minimum cost. Each cut divides activities into two non-overlapping partitions with an operator that could be sequence cut, exclusive choice cut, parallel cut, or loop cut. Then, the event log is projected onto the partitions to create new sub-event logs. Recursively, for each sub-event log, the algorithm searches for the cut with minimum cost. The recursion continues until reaching base cases. The algorithm generates a process tree that could easily be converted to a Petri net.
In our project, we discover white-box interpretable process models. In the Tandem partner project, desirable and undesirable event logs are used to train machine learning models that classify the traces as desirable or undesirable. The trained machine learning models classify the traces with high accuracy but do not describe the control flow of the process from beginning to end. An interesting question for our future research is investigating the possibility of discovering white-box interpretable process models using machine learning techniques.
Cooperation
References
[1]: Augusto, A., Conforti, R., Dumas, M., La Rosa, M., Maggi, F.M., Marrella, A., Mecella, M. and Soo, A., 2018. Automated discovery of process models from event logs: review and benchmark. IEEE transactions on knowledge and data engineering, 31(4), pp.686-705.
[2]: van der Aalst, W.M., 2016. Process mining: data science in action. Springer.
[3]: Buijs, J.C., van Dongen, B.F. and van der Aalst, W.M., 2014. Quality dimensions in process discovery: The importance of fitness, precision, generalization and simplicity. International Journal of Cooperative Information Systems, 23(01), p.1440001.
Project publications
- Artelt, André, Valerie Vaquet, Riza Velioglu, Fabian Hinder, Johannes Brinkrolf, Malte Schilling, and Barbara Hammer (2021). ‘‘Evaluating robustness of counterfactual explanations’’. In: IEEE Symposium Series on Computational Intelligence (SSCI).
- Eichenberger, Christian, Moritz Neun, Henry Martin, Pedro Herruzo, Markus Spanring, Yichao Lu, Sungbin Choi, Vsevolod Konyakhin, Nina Lukashina, Aleksei Shpilman, et al. (2022). ‘‘Traffic4cast at neurips 2021-temporal and spatial few-shot transfer learning in gridded geo-spatial processes’’. In: NeurIPS 2021 Competitions and Demonstrations Track.
- Norouzifar, Ali and Wil M. P. van der Aalst (2023). ‘‘Discovering Process Models that Support Desired Behavior and Avoid Undesired Behavior’’. In:Proceedings of the 38th ACM/SIGAPP Symposium on Applied Computing, SAC 2023, Tallinn, Estonia, March 27-31, 2023. Ed. by Jiman Hong, Maart Lanperne, Juw Won Park, Tomás Cerný, and Hossain Shahriar. ACM, pp. 365–368. doi: 10.1145/3555776.3577818. url:https://doi.org/10.1145/3555776.3577818.
- Norouzifar, Ali and Wil M. P. van der Aalst (2024). ‘‘Leveraging Desirable and Undesirable Event Logs in Process Mining Tasks’’. In: DataNinja sAIOnARA 2024 Conference abs/2408.17316, pp. 32–35. doi: 10.11576/dataninja-1164. url: https://doi.org/10.11576/dataninja-1164.
- Norouzifar, Ali, Marcus Dees, and Wil M. P. van der Aalst (2024). ‘‘Imposing Rules in Process Discovery: An Inductive Mining Approach’’. In: Research Challenges in Information Science – 18th International Conference, RCIS 2024, Guimarães, Portugal, May 14-17, 2024, Proceedings, Part I. Ed. by João Araújo, Jose Luis de la Vara, Maribel Yasmina Santos, and Said Assar. Vol. 513. Lecture Notes in Business Information Processing. Springer, pp. 220–236. doi: 10.1007/978-3-031-59465-6\_14. url: https://doi.org/10.1007/978-3-031-59465-6%5C_14.
- Norouzifar, Ali, Humam Kourani, Marcus Dees, and Wil M. P. van der Aalst (2024). ‘‘Bridging Domain Knowledge and Process Discovery Using Large Language Models’’. In: Proceedings of the 22nd International Conference on Business Process Management, in print.
- Norouzifar, Ali, Majid Rafiei, Marcus Dees, and Wil M. P. van der Aalst (2024a). ‘‘An Framework for Explainable Process Variant Analysis on Continuous Features’’. submitted.
- Norouzifar, Ali, Majid Rafiei, Marcus Dees, and Wil M. P. van der Aalst (2024b). ‘‘Process Variant Analysis Across Continuous Features: A Novel Framework’’. In: Enterprise, Business-Process and Information Systems Modeling – 25th International Conference, BPMDS 2024, and 29th International Conference, EMMSAD 2024, Limassol, Cyprus, June 3-4, 2024, Proceedings. Ed. by Han van der Aa, Dominik Bork, Rainer Schmidt, and Arnon Sturm. Vol. 511. Lecture Notes in Business Information Processing. Springer, pp. 129–142. doi: 10.1007/978-3-031-61007-3\_11. url: https://doi.org/10.1007/978-3-031-61007-3%5C_11.
- Özdemir, Özgür, Emre Salih Akın, Rıza Velioğlu, and Tuğba Dalyan (2022). ‘‘A comparative study of neural machine translation models for Turkish language’’. In: Journal of Intelligent & Fuzzy Systems.
- Velioglu, Riza, Petra Bevandic, Robin Chan, and Barbara Hammer (2024). ‘‘Clothify: Redefining Fashion E-Commerce Photography with Virtual Try-Off and Diffusion Models’’. submitted to: IEEE/CVF international conference on computer vision.
- Velioglu, Riza, Robin Chan, and Barbara Hammer (2024). ‘‘FashionFail: Addressing Failure Cases in Fashion Object Detection and Segmentation’’. In: International Joint Conference on Neural Networks (IJCNN). IEEE.
- Velioglu, Riza, Jan Philip Göpfert, André Artelt, and Barbara Hammer (2022). ‘‘Explainable Artificial Intelligence for Improved Modeling of Processes’’. In: Intelligent Data Engineering and Automated Learning (IDEAL).