The faces behind project RoSe
Adaptive Helpers: Devices that Think and Support
Every person is different. Some are tall, some are short, some have more or less muscle, and even bones vary from person to person. These differences are very important for us in the RoSe project, because we work with sensors that are worn directly on the body and measure signals from it—these are called biosignals. You might be familiar with this from a doctor using an ECG to check your heart.
Based on these signals, we are developing two devices: First, we are working on a supportive frame worn on the elbow. This so-called exoskeleton detects the muscles’ movements and helps the arm move. It’s useful for people who frequently lift heavy loads.
Second, we are creating an insole for shoes, with built-in pressure sensors. This device constantly measures how much weight is being put on the foot or leg while walking. It can alert people after surgery or injury, protecting them from overloading the affected limb.
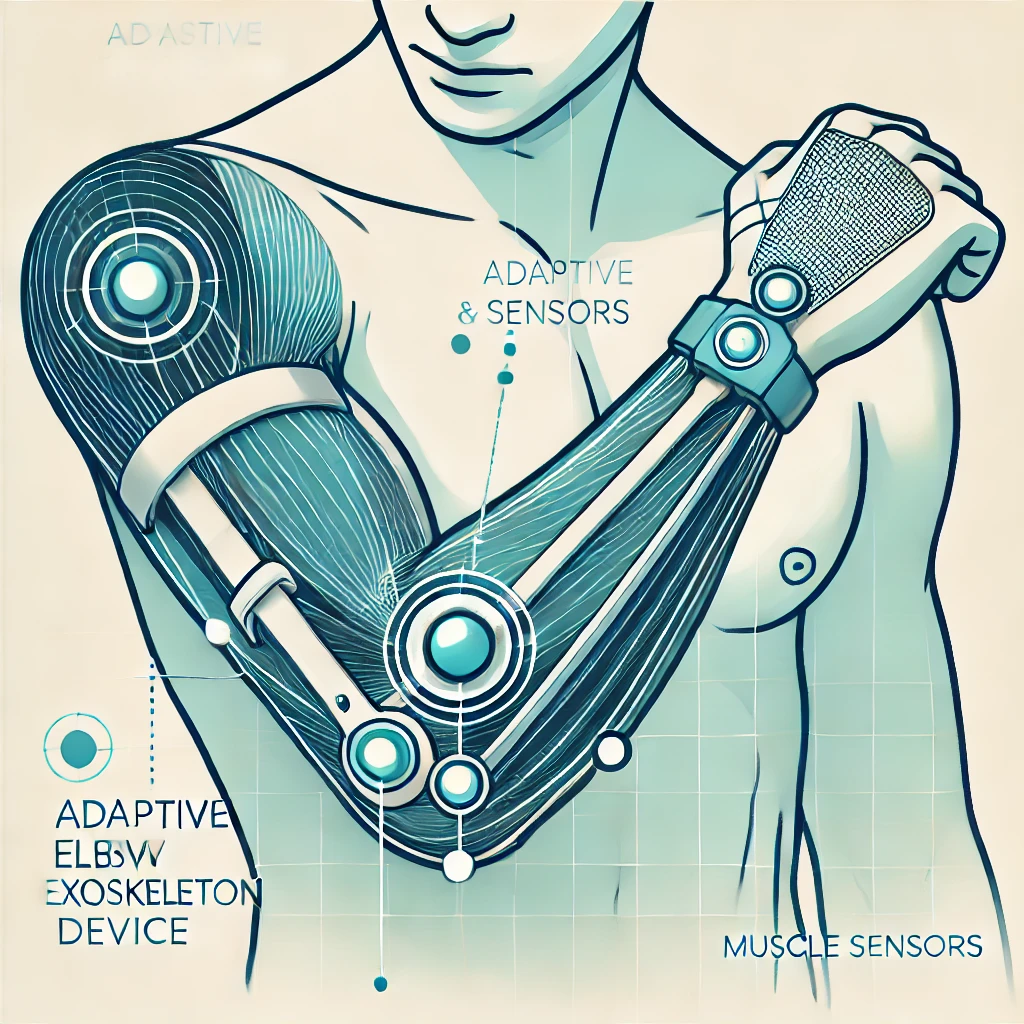
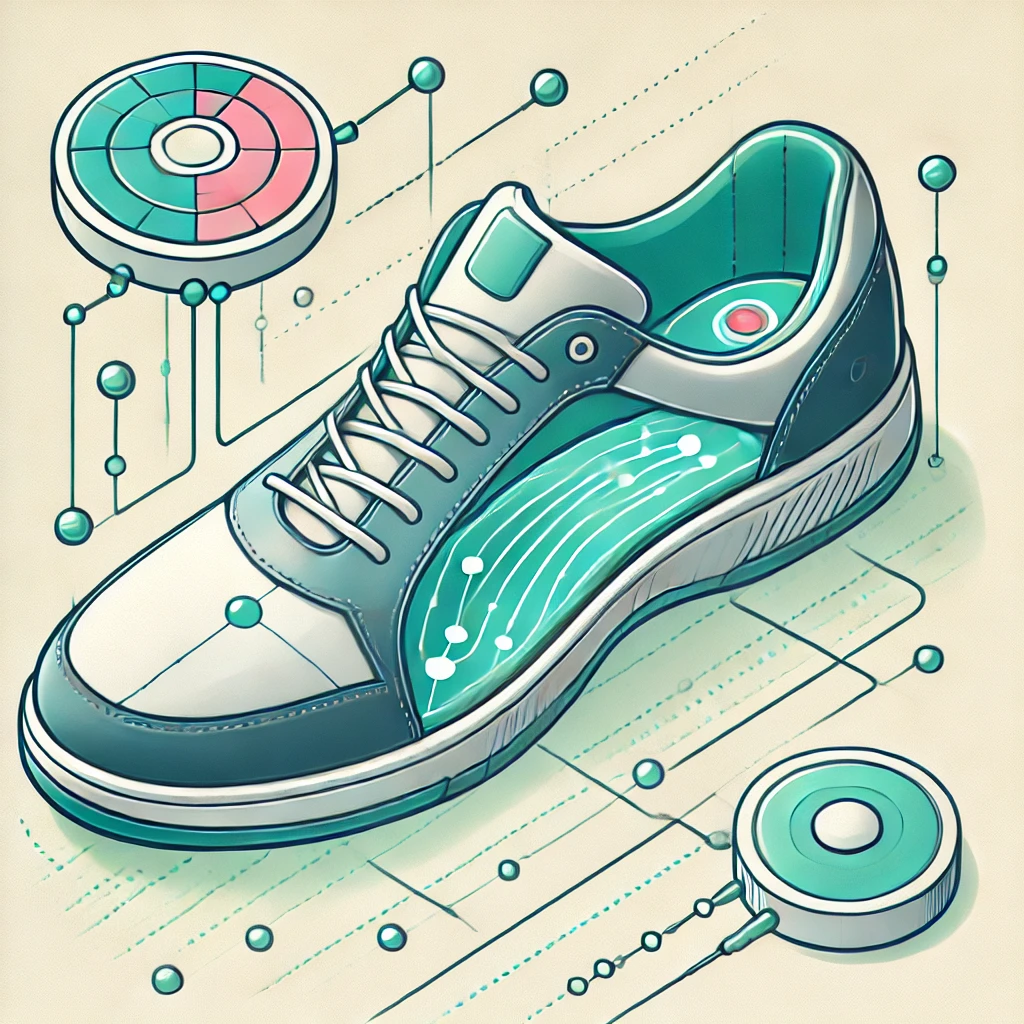
But since every person is different, we can’t create a custom device for each individual. That would be too expensive and take too much time. Instead, we design these two devices to fit as many people as possible. We also program the devices to adjust to each person and always provide good results. We are researching how the information we learn from one person’s feet and arms can be applied to others.
For our devices, we use knowledge from doctors, for example, about muscles, and we also use artificial intelligence (AI). AI learns from lots of data and performs calculations, like how fast the arm moves when certain muscles contract. Combining both approaches helps us achieve more accurate results. In summary, we are developing devices that support people and work well for everyone.
Cooperation
Project Publications
- Grimmelsmann, Nils, Malte Mechtenberg, Wolfram Schenck, Hanno Gerd Meyer, and Axel Schneider (Aug. 2023). ‘‘sEMG-based prediction of human forearm movements utilizing a biomechanical model based on individual anatomical/ physiological measures and a reduced set of optimization parameters’’. In: PLOS ONE 18.8, pp. 1–28. doi:10.1371/journal.pone.0289549. url: https://doi.org/10.1371/journal.pone.0289549.
- Grimmelsmann, Nils, Malte Mechtenberg, Markus Vieth, Barbara Hammer, and Axel Schneider (2024). ‘‘Prediction of Intermuscular Co-contraction Based on the sEMG of Only One Muscle With the Same Biomechanical Direction of Action’’. en. In: DataNinja sAIOnARA Conference, pp. 47–49. doi: 10.11576/DATANINJA-1168. url: https://biecoll.ub.uni-bielefeld.de/index.php/dataninja/article/view/1168.
- Grimmelsmann, Nils, Malte Mechtenberg, Markus Vieth, Alexander Schulz, Barbara Hammer, and Axel Schneider (2024). ‘‘Predicting the Level of Co-Activation of One Muscle Head from the Other Muscle Head of the Biceps Brachii Muscle by Linear Regression and Shallow Feedforward Neural Networks.’’ In: In Proceedings of the 17th International Joint Conference on Biomedical Engineering Systems and Technologies (BIOSTEC 2024).
- Leserri, David, Nils Grimmelsmann, Malte Mechtenberg, Hanno Gerd Meyer, and Axel Schneider (Mar. 2022). ‘‘Evaluation of sEMG Signal Features and Segmentation Parameters for Limb Movement Prediction Using a Feedforward Neural Network’’. In: Mathematics 10.6, p. 932. issn: 2227-7390. doi: 10.3390/math10060932. url: http://dx.doi.org/10.3390/math10060932.
- Vieth, Markus (2024). ‘‘Nonlinear Prediction in a Smart Shoe Insole’’. en. In: DataNinja sAIOnARA Conference, pp. 50–52. doi: 10.11576/dataninja-1169. url: https://biecoll.ub.uni-bielefeld.de/index.php/dataninja/article/view/1169.
- Vieth, Markus, Nils Grimmelsmann, Axel Schneider, and Barbara Hammer (2022). ‘‘Efficient Sensor Selection for Individualized Prediction Based on Biosignals’’. In: Intelligent Data Engineering and Automated Learning — IDEAL 2022. Ed. by Hujun Yin, David Camacho, and Peter Tino. Cham: Springer International Publishing, pp. 326–337. isbn: 978-3-031-217531.
- Vieth, Markus, Nils Grimmelsmann, Axel Schneider, and Barbara Hammer (2024). ‘‘Similarity-Based Zero-Shot Domain Adaptation for Wearables’’. In: ESANN 2024 Proceedings. Bruges (Belgium) and online event. doi:10.14428/esann/2024.ES2024-123.
- Vieth, Markus, Alexander Schulz, and Barbara Hammer (2023). ‘‘Extending Drift Detection Methods to Identify When Exactly the Change Happened’’. In: Advances in Computational Intelligence. Ed. by Ignacio Rojas, Gonzalo Joya, and Andreu Catala. Cham: Springer Nature Switzerland, pp. 92–104. isbn: 978-3-031-43085-5.