The faces behind project NireHApS
Machine Learning without Coding
Introduction
The aim of the project is a design-space exploration of embedded hardware platforms, which on the one hand allow the resource-efficient execution of spiking neural networks (SNNs), but on the other hand, also allow the possibility of adaptation (i.e., online learning). The exploration of neuromorphic accelerators includes reconfigurable hardware platforms (Field Programmable Gate Arrays (FPGAs)) and GPU-based embedded devices (such as NVIDIA Jetson).
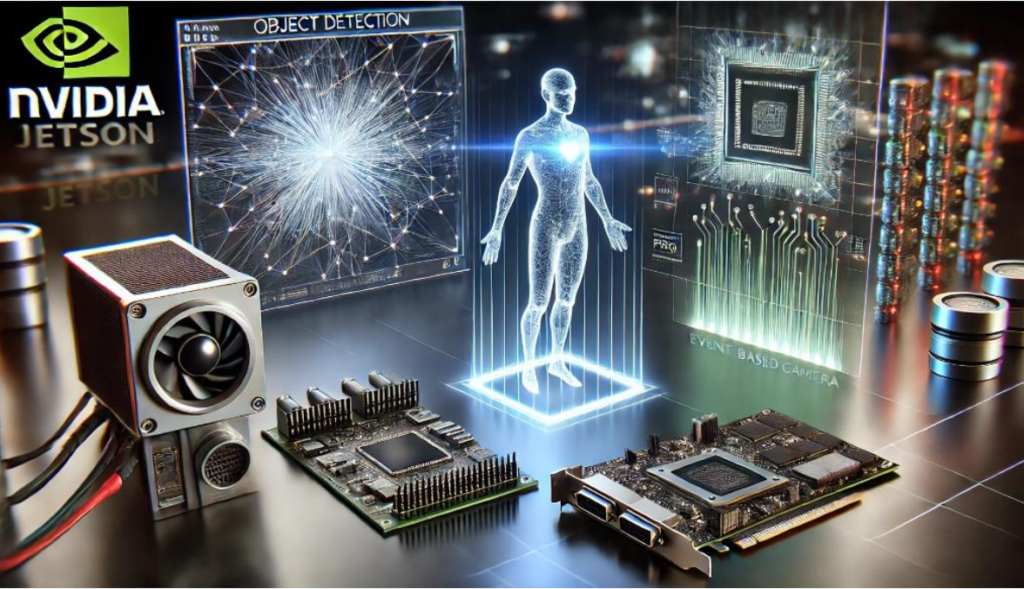
The foundation of this project is an integrated design process that utilizes a backend-independent representation to describe the SNN architecture, learning algorithm, and models of neurons and synapses. This process automatically maps SNNs to configurable hardware architectures.
This project focuses on two sub-projects: exploring computer vision design spaces and analyzing resource-efficient implementations of biologically inspired SNN models using two different embedded platforms with integrated spiking cameras, FPGA (explored by Bielefeld University) and Jetson devices (explored by Hochschule Bielefeld). Applications include tasks like multi-object detection, active object counting, and deployment on embedded devices with event-based cameras.
Let’s Explore How It’s Achieved
This research explored various aspects of SNNs, including network architecture, learning algorithms, and neuron models. These are all essential for determining the parameters required for exploring hardware platforms. The work unfolded in a series of phases, integrating theoretical studies, practical application development, and hardware implementation.
SNNs rely on numerous parameters that must be carefully balanced to achieve accurate results. Existing state-of-the-art SNN simulators, such as Brian2 and NEST, have been primarily designed for exploring brain functionalities and neuronal dynamics. However, they present significant usability challenges. Adding new functionalities often requires working in low-level programming languages like C++ and integrating the modifications into the simulator’s codebase. Moreover, some simulators require domain-specific languages, such as NESTML for NEST and NMODL for NEURON, which are text-based and require extensive coding, even for minor updates. These complexities pose a significant hurdle, especially for early-stage researchers and students, who spend considerable time learning how to operate these simulators instead of focusing on understanding SNN behavior and adapting their features for real-time applications. To address these challenges, we developed RAVSim, a runtime analysis and visualization simulator for SNNs. RAVSim allows users to make real-time changes to a simulation and immediately observe their effects on the model’s behavior. This feature not only accelerates simulations but also facilitates tasks like design, prototyping, and parameter tuning. RAVSim simplifies the analysis and visualization of SNN models by enabling users to adjust input parameters dynamically and explore complex structural features more intuitively.
The initial focus was on evaluating the complexity of different neuron models to identify which properties—such as refractory times, adaptive thresholds, and membrane potential, are essential for hardware implementation. We conducted a thorough analysis of SNN models to simulate neuron behavior while also assessing their performance for hardware implementation. This evaluation focused on key factors such as resource utilization, speed, and power consumption. Hardware Description Languages (HDLs) were used for the hardware design, with simulations run in Vivado. Emulation experiments were then conducted on the Basys3 FPGA board to validate our findings. To gain hands-on experience with SNN behavior, we implemented Binary Neural Associative Memory (BiNAM) using the explored neuronal models. We implemented the core modules of BiNAM for hardware designing, and we emulated these modules on FPGA platforms (Basys3 and Zybo-Z7) for real-time hardware deployment. We also developed and implemented SNNs that are capable of imitating the behavioral characteristics of biological neural networks. To achieve this, we designed the fundamental modules. The neuron, Address-Event Representation (AER) module for data communication and a training algorithm called STDP to provide functionality to the neural network. We implemented an SNN model using these modules for pattern recognition application, which was emulated on the Basys3 FPGA development board to demonstrate the accuracy of the digital system’s operation.
One of the main goals of this project is to integrate event-based cameras with the explored hardware platforms. To effectively utilize the data generated by these cameras, we have analyzed various techniques for processing event-based camera data and designing SNNs specifically for tasks like object detection and tracking.
RAVSim
SNNs rely on many parameters, and achieving accurate results requires precise balancing of these parameters. While state-of-the-art SNN simulators like Brian2 and NEST have been developed primarily for exploring brain functionality and neuronal dynamics, they are not particularly user- friendly. Adding new features to these simulators often requires low-level programming, such as writing code in C++, and integrating it into the existing simulator code. Additionally, some simulators require the use of domain-specific languages, such as NESTML for NEST or NMODL for NEURON. These tools are based on textual programming languages, meaning that even simple updates, like adjusting a single parameter value, necessitate lengthy code and can be time- consuming. For early-stage researchers and students, this makes it challenging to quickly understand the behavior of SNNs and exploit their potential for real-time applications.
To address these challenges, we developed RAVSim, a runtime analysis and visualization tool for SNNs. RAVSim allows users to make real-time adjustments to simulations and instantly observe the behavior of the model. This tool accelerates existing simulations and assists with designing, prototyping, and fine-tuning parameters. It not only simplifies the process of analyzing and visualizing SNN models but also helps users better understand the complex structures of these models. With RAVSim, users can visualize spike detection based on input currents and continuous signal values through a mixed-signal plot. The current version of RAVSim supports three different connectivity schemes for neuron communication: i == j (a network where each neuron connects to itself), a fully connected network, and i ≠j (a network where each neuron connects to others except itself).
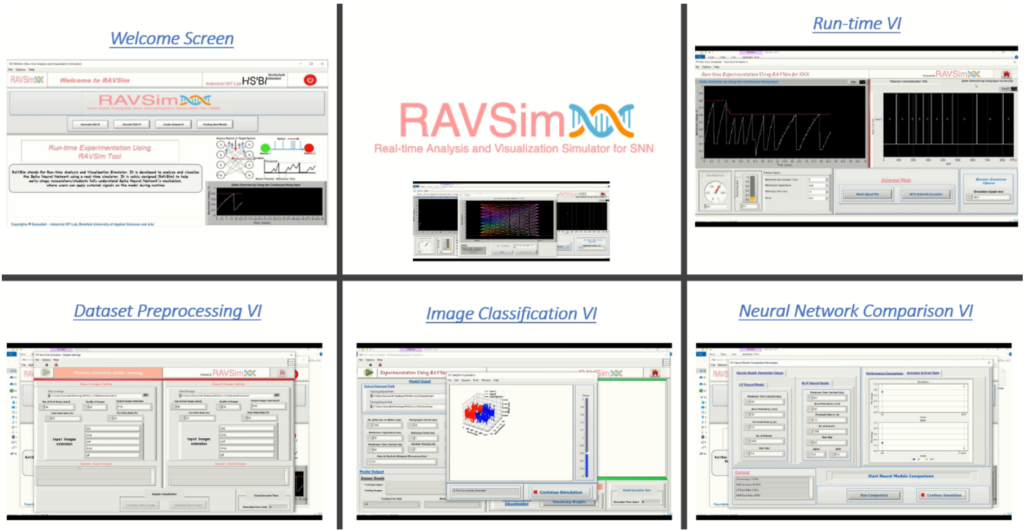
We verified the outputs from RAVSim by comparing them to results obtained using the Brian2 simulator, using the same input parameters. Our results show that RAVSim is faster than Brian2, because of its efficient implementation and parallel processing capabilities. RAVSim is built entirely with LabVIEW, without relying on external libraries. LabVIEW is well-suited for runtime simulations and offers fast computation. In contrast, Brian2 uses a Python interpreter, which can be slow for more complex models.
Recently, we released RAVSim version 1.2, an upgraded version of the simulator designed specifically for classification tasks. This new version offers improved performance and functionality, further enhancing its utility for the SNN community.
BiNAM
We developed a simplified spiking binary associative memory (BiNAM) model to evaluate how the complexity of neuron or synapse models impacts application performance. Associative memory is a type of mapping memory that maps input patterns to output patterns. The spiking variant of BiNAM is inspired by the binary associative memory model introduced by Palm (1980). It consists of a single layer of neurons, with each neuron corresponding to one output element. The network architecture is implemented using various neuron models, such as Leaky Integrate- and-Fire (LIF), Hodgkin-Huxley (HH), and Izhikevich (IZH). In this model, “1s” or set bits in the input vector are encoded as single spikes. Output decoding interprets spikes within specific time windows as set bits in the corresponding output pattern. A pre-trained storage matrix 𝑀𝑥𝑦 determines connectivity: a synapse is established between input 𝑥 and output neuron 𝑦 when 𝑀𝑥𝑦 = 1. The inputs, weighted by their synaptic connections, are summed and compared against a threshold. If it exceeds the threshold, the neuron fires; otherwise, it returns to its resting potential.
Unlike classical associative memory, spiking BiNAM introduces additional neuron parameters that must be optimized for effective performance. This optimization is achieved through multidimensional parameter sweeps. Using the Brian2 simulator, we implemented the SNN model and generated sparse input and output patterns to achieve reasonable storage capacity. Key parameters, such as thresholds and synaptic weights, were tuned through experiments to assess storage efficiency across varying conditions.
BiNAM can also serve as a training algorithm for spiking neural networks in associative learning tasks. Foundational BiNAM modules have been implemented using hardware description languages (HDLs) and emulated on FPGA platforms (Basys3 and Zybo-Z7) for edge-device deployment. These implementations demonstrate the model’s ability to associate patterns effectively in resource-constrained environments.
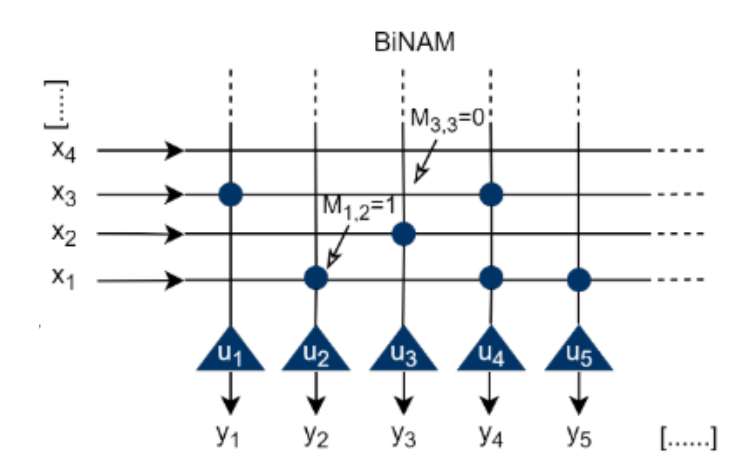
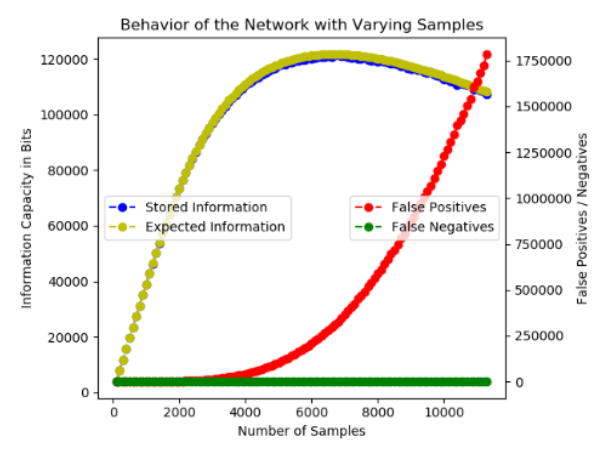
Pattern Recognition
We developed and implemented SNNs to replicate the behavioral characteristics of biological neural networks. To achieve this, we designed three core modules:
- Neuron Simulation Module: This module models the properties, characteristics, and behavior of a single neuron using a mathematically accurate yet computationally efficient approach. The model is optimized for implementation on FPGA platforms.
- Address-Event Representation (AER): Based on the work of Mahowald and Mead (1991), this module manages the flow of information between neurons in the network, enabling efficient communication.
- Spike-Timing-Dependent Plasticity (STDP) Training Algorithm: This module provides the learning functionality for the neural network, allowing it to adapt based on input patterns.
These modules were interconnected to simulate SNNs with the desired functionality. Our modular design ensures flexibility and scalability, making it suitable for simulating various types of SNNs. The modules were implemented using a combination of synchronous flip-flops and combinational logic driven by a 100 MHz clock. The digital system was described using hardware description languages (HDLs) and simulated with Xilinx Vivado tools.
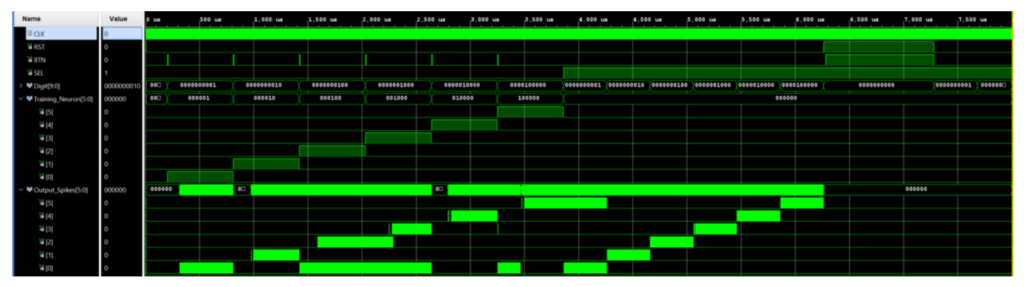
To demonstrate the practical application of this system, we implemented an SNN model for pattern recognition. This model was emulated on the Basys3 FPGA development board, showcasing the accuracy and efficiency of the digital system.
Event-Based Cameras and SNN-Based Object Recognition and Detection
Event-based cameras, such as the DVS, are bio-inspired vision sensors that output pixel-level brightness changes (events) instead of traditional intensity frames. Unlike conventional cameras, event cameras operate asynchronously, sampling light based on scene dynamics rather than at fixed intervals. This design offers significant advantages, including an extremely high dynamic range (~140 dB), microsecond-level latency, and no motion blur. Due to their unique output, novel processing methods are required to unlock their full potential.
Our Approach with the DAVIS 346 Camera
We utilized the DAVIS 346 event-based camera, a 346×260 pixel DVS sensor with an integrated active pixel frame sensor and multi-camera time synchronization. Our experiments focused on SNNs for various recognition tasks, inspired by the unique capabilities of event-based cameras.
1. Object Recognition Using N-MNIST Dataset
The N-MNIST dataset provides event-based representations of handwritten digits. For this task, we designed a YOLO-inspired SNN architecture to classify and detect objects, aiming to maintain simplicity while maximizing performance.
Network Architecture and Methodology
- Structure: The network featured convolutional layers combined with Leaky Integrate- and-Fire (LIF) neurons.
- Unified Detection and Classification: The network performed object detection and classification simultaneously by processing each input frame iteratively.
- Result Aggregation: Outputs from all frames were stacked, and the maximum voltage for each class was taken as the final result.
- Performance Optimization: Training techniques included careful hyperparameter tuning and ensuring robust handling of asynchronous data.
Results
- Object Detection Accuracy: 99%
- Classification Accuracy: 85%
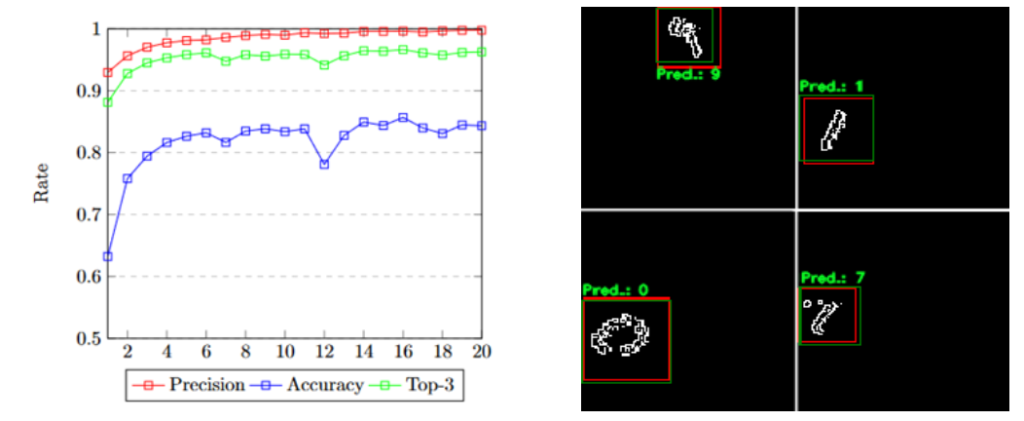
2. Gesture Recognition Using DVS128 Gesture Dataset
The DVS128 Gesture dataset is a neuromorphic video dataset for gesture recognition. We experimented with different lib raries and cameras to train SNNs for this task.
Two Approaches
- Using the DAVIS 346 Camera and Norse Library:
- The event-based data from the DAVIS 346 was processed directly.
- The high temporal resolution of the DVS (1 ÎĽs) allowed for precise gesturerepresentation.
- Training included:
- Batch Normalization: To standardize input distribution.
- Data Augmentation: To enhance model robustness.
- Randomization: Between epochs to prevent overfitting.
- Layer Freezing: Freezing parts of the network after initial training forstability.
- Depth Tuning: Exploring the depth of the architecture to balanceperformance and complexity.
- Using an RGB Camera and snnTorch Library:
- RGB camera outputs were preprocessed to emulate event-based input.
- Frames were downsampled to a temporal resolution of 20–30 fps to align withneuromorphic data formats.
Results
- Classification Accuracy: 93.75%
- Gesture Recognition Accuracy: 66%
- Localization Accuracy: ~100%
These results highlighted the superior temporal resolution of event-based cameras for capturing dynamic gestures and the effectiveness of SNNs for asynchronous data processing.
3. Real-Time Person Detection and Counting
We proposed a novel architecture combining the strengths of SNNs and transfer learning for real- time person detection and counting using event-based cameras.
Pipeline Components
- Object Detection: The system continuously monitors for motion events in the environment.
- Transfer Learning: Pre-trained CNN weights are adapted to SNNs, enabling efficient event- driven processing.
- Real-Time Human Recognition and Tracking: Detected individuals are localized and tracked.
- Feature Extraction and Run-Time Analysis: Captures and analyzes data for applications such as behavioral studies and live monitoring.
Implementation Details
- Edge Deployment: The architecture was implemented on Nvidia edge devices, leveraging multi-core processing for real-time analytics.
- Spike Train Dataset: Maintained a dataset of spike trains to record critical information about recognized objects for further analysis.
- Comparison: The system was compared with traditional cameras, demonstrating the advantages of event-based processing, especially in dynamic or low-light environments.
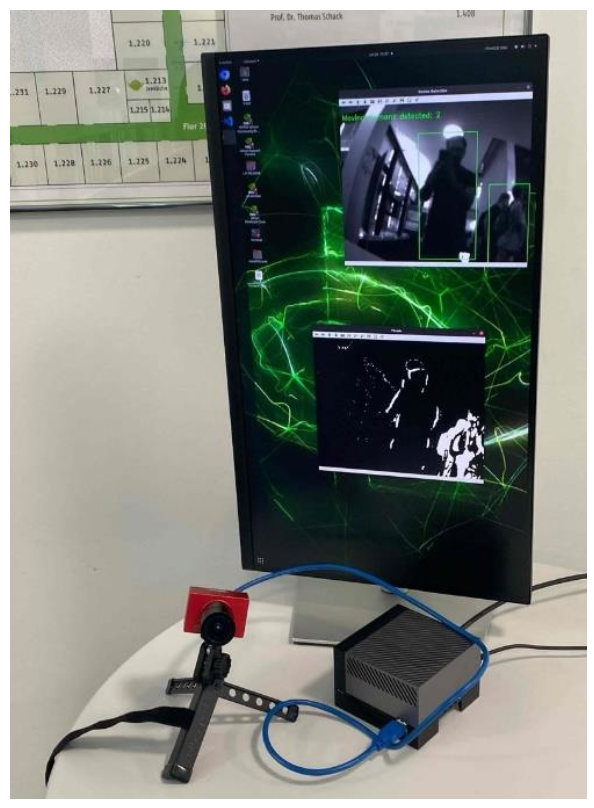
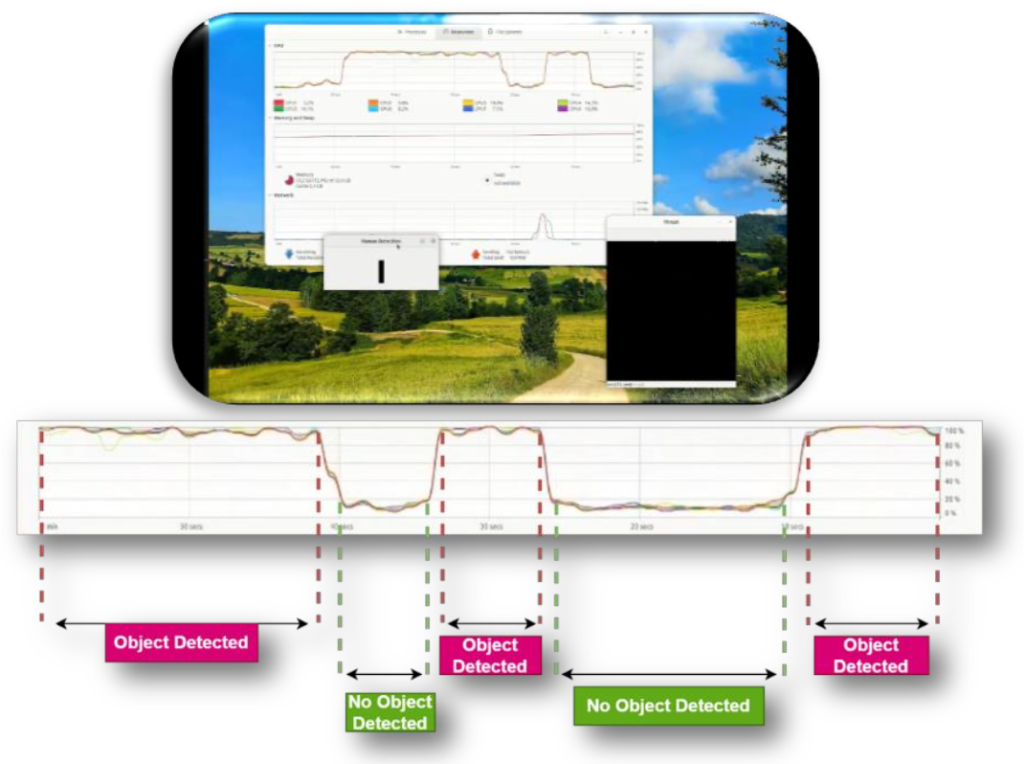
4. FPGA Integration
To enhance performance and scalability, we interfaced event-based cameras with FPGAs (https://github.com/ShaminiKoravuna/Kria_PYNQ_EBC), specifically Kria KV260 and PYNQ-Z2. The goal is to deploy high-performance neuromorphic applications using high-level synthesis (HLS).
Current Efforts
- Developing efficient hardware pipelines for real-time detection and analysis.
- Optimizing SNN architectures for FPGA implementation to balance latency, power consumption, and accuracy.
Conclusion
To support this research, RAVSim was developed as a tool for analyzing and visualizing the behavior of SNNs in real time. RAVSim allows users to interact with models, observe their dynamic behavior, and make adjustments at runtime. This eliminates the need for time-consuming code- based experiments, offering a faster, more intuitive way to design and understand SNNs. It is designed to be user-friendly and accessible, especially for students and early-stage researchers. The tool features graphical outputs that make complex models easier to comprehend and allows for quicker retrieval of parametric values.
RAVSim v1.2 introduced an upgraded version of the tool, which includes a faster and more accurate image classification algorithm based on SNNs. This version enables users to interact with different RGB image datasets and adjust parametric values seamlessly. Specifically, the updated tool was used to classify masked and non-masked face images within a wild RGB dataset. In RAVSim v1.1, users can create custom datasets from downloaded images without the need for third-party APIs, facilitating model training and testing. In one experiment, using 1000 neurons in hidden layers, the model achieved an accuracy of 91.8% on a CPU in just 10 minutes. A key challenge faced was reducing image classification errors to make SNNs comparable to deep neural networks in recognizing distinct classes.
The results obtained using BiNAM demonstrate the performance of the SNN variant compared to traditional models, with the storage capacity being directly proportional to the number of samples. Among the various neuronal models tested, the one utilizing LIF neurons outperformed the more complex models. The storage performance of the system was evaluated by adjusting the threshold and weights, using default parameters for inputs and outputs. It was observed that smaller weights (below the default value) caused information loss, leading to false negatives, while larger weights resulted in an increase in false positives. The optimal performance was achieved when the weight value was adjusted to minimize both false negatives and false positives.
To facilitate the emulation of the SNN on edge AI devices, the model was implemented on FPGA platforms. A modular approach was adopted to make the emulation process simple, efficient, flexible, and scalable. The system is built with three core, independent blocks: the neuron, AER Communication Bus, and STDP learning system. These modules can be easily connected and replicated to emulate neural networks with varying sizes, from just two neurons to thousands, depending on available resources.
For the object detection task using N-MNIST dataset, the SNN was trained for 20 epochs with a batch size of 256. The model achieved a detection accuracy of 99%, with stable performance throughout the training process. The classification accuracy was 85%, showing a notable difference between the detection and classification tasks as they balanced the network.
For the DVSGesture application, we explored different libraries, models, and training techniques to develop SNNs capable of successfully classifying and localizing gestures in neuromorphic video feeds. With the Norse library, we learned that choosing the correct number of convolutional layers and batch normalization was critical for improving model performance. To address overfitting during training, we randomized the dataset after each epoch and implemented a technique to freeze the shared layers during localization training. This approach ensured that classification accuracy was not compromised by localization tasks. For snnTorch, although the model achieved 93.75% accuracy on the training data, it did not perform as well on video data converted into event-based format.
In the Person Detection and Counting application, we proposed a novel architecture using neuromorphic computing to detect and analyze human presence in real-time with event-based cameras. This dynamic system integrated several strategies, including object detection, transfer learning with SNN, human recognition, localization, tracking, feature extraction, multi-core processing, and real-time analysis. The system continuously monitors the environment for motion events, which trigger the detection algorithms. The transfer learning approach combines pre- trained CNN weights with SNNs, enabling event-driven processing while simulating the behavior of spiking neurons, thus creating a more efficient and adaptive detection system.
Through the design and implementation of these applications, we gained valuable experience in developing SNNs for edge AI applications. The DAVIS-346 camera provided crucial insights into working with event-based data, particularly in real-time high-speed computer vision tasks. The insights gained contribute to the development of trustworthy AI systems that process data on edge devices. Additionally, the use of the RAVSim runtime simulator helped deepen our understanding of SNN behavior, enabling the design and simulation of complex models with precise parametric values.
Additional resources
The research results presented during the project have been disseminated through the following platforms:
Code Repositories
Sanaullah: https://github.com/Rao-Sanaullah?tab=repositories
Shamini: https://github.com/ShaminiKoravuna?tab=repositories
RAVSim
Project Webpage: https://rao-sanaullah.github.io/RAVSim-webpage/
LabVIEW’s official website: https://www.ni.com/en/support/downloads/tools-network/download.run- time-analysis-and-visualization-simulator–ravsim-.html
Quick start guide: Compatibility requirements, setup instructions, and user manual can be accessed at https://github.com/Rao-Sanaullah/RAVSim/tree/main/User-Manual
Recorded Demonstrations
Multi-Object Detection Application: Demonstrations and detailed results can be accessed at https://rao-sanaullah.github.io/neurocomputing_application/
RAVSim: Demonstrations and detailed results can be accessed at: https://rao-sanaullah.github.io/RAVSim-webpage/
Cooperation
Project Publications
Journals
- Sanaullah, Shamini Koravuna, Ulrich RĂĽckert, and Thorsten Jungeblut. “Evaluation of Spiking Neural Nets- Based Image Classification Using the Runtime Simulator RAVSim.” International Journal of Neural Systems (2023): pp.2350044-2350044.
- Sanaullah, Shamini Koravuna, Ulrich RĂĽckert, and Thorsten Jungeblut. “Exploring Spiking Neural Networks: A Comprehensive Analysis of Mathematical Models and Applications.” International Journal of Frontiers in Computational Neuroscience 17 (2023).
- Hasina Attaullah, Sanaullah, and Thorsten Jungeblut. “Analyzing Machine Learning Models for Activity Recognition Using Homomorphically Encrypted Real-World Smart Home Datasets: A Case Study.” International Journal of Applied Sciences 14.19 (2024): 9047.
Conferences
- Sanaullah, Shamini Koravuna, Ulrich RĂĽckert, and Thorsten Jungeblut. “SNNs Model Analyzing and Visualizing Experimentation using RAVSim.” In International Conference on Engineering Applications of Neural Networks (EANN), 2022.
- Sanaullah, Shamini Koravuna, Ulrich RĂĽckert, and Thorsten Jungeblut. “Design- Space Exploration of SNN Models using Application-Specific Multi-Core Architectures.” In International Conference on Neuro-Inspired Computing Elements (NICE), 2023.
- Sanaullah, Shamini Koravuna, Ulrich RĂĽckert, and Thorsten Jungeblut. “Streamlined Training of GCN for Node Classification with Automatic Loss Function and Optimizer Selection.” In International Conference on Engineering Applications of Neural Networks (EANN), 2023.
- Shamini Koravuna, Sanaullah, Thorsten Jungeblut, and Ulrich RĂĽckert. Digit Recognition Using Spiking Neural Networks on FPGA. In International Work- Conference on Artificial Neural Networks (IWANN), 2023.
- Sanaullah and Thorsten Jungeblut.” Analysis of MR Images for Early and Accurate Detection of Brain Tumor using Resource Efficient Simulator Brain Analysis”. In International Conference on Machine Learning and Data Mining (MLDM) 2023.
- Sanaullah, Amanullah, Kaushik Roy, Jeong-A. Lee, Son Chul-Jun, and Thorsten Jungeblut. “A Hybrid Spiking- Convolutional Neural Network Approach for Ad- advancing High-Quality Image Inpainting.” In International Conference on Computer Vision – workshop: PerDream (ICCV-PerDream), 2023.
- Sanaullah, Shamini Koravuna, Ulrich RĂĽckert, and Thorsten Jungeblut. “Transforming Event-Based into Spike-Rate Datasets for Enhancing Neuronal Behavior Simulation to Bridging the Gap for SNNs” In International Conference on Computer Vision – workshop: PerDream (ICCV-PerDream), 2023.
- Sanaullah, Shamini Koravuna, Ulrich RĂĽckert, and Thorsten Jungeblut. “A Novel Spike Vision Approach for Robust Multi-Object Detection using SNNs.” In International Workshop on Novel Trends in Data Science (NTDS), 2023.
- Sanaullah, Kaushik Roy, Ulrich RĂĽckert, and Thorsten Jungeblut. “A Hybrid Spiking-Convolutional Neural Network Approach for Advancing Machine Learning Models.” In International Conference on Northern Lights Deep Learning (NLDL), 2024. (Best Presenter)
- Sanaullah, Shamini Koravuna, Ulrich RĂĽckert, and Thorsten Jungeblut. “Advancements in Neural Network Generations.” In International Conference on Shaping Trustworthy AI: Opportunities, Innovation, and Achievements for Reliable Approaches (sAIOnARA), 2024. (2nd Best Award)
- Sanaullah, Hasina Attaullah, Thorsten Jungeblut. “Trade-offs Between Privacy and Performance in Encrypted Dataset using Machine Learning Models.” In International Conference on shaping trustworthy AI: Opportunities, Innovation, and Achievements for Reliable Approaches (sAIOnARA), 2024.
- Sanaullah, Shamini Koravuna, Ulrich RĂĽckert, and Thorsten Jungeblut. “A Spike Vision Approach for Multi-Object Detection and Generating Dataset Using Multi-Core Architecture on Edge Device.” In International Conference on Engineering Applications of Neural Networks (EANN), 2024.Sanaullah, Hasina Attaullah, Thorsten Jungeblut. “Encryption Techniques for Privacy-Preserving CNN Models Performance and Practicality in Urban AI Applications.” In International Conference on 32nd ACM SIGSPATIAL – Workshop on Advances in Urban-Al 2024.
- Hasina Attaullah, Sanaullah, and Thorsten Jungeblut. “FL-DL: Fuzzy Logic with Deep Learning, Hybrid Anomaly Detection and Activity Prediction in Smart Homes Data-Sets.” In International Conference and Symposium on Computational Intelligence and Informatics (CINTI), 2024.
- S. Koravuna, Sanaullah, T. Jungeblut, and U. Rückert. “Spiking Neural Network Models Analysis on Field Programmable Gate Arrays”. In International Conference on Intelligent and Innovative Computing Applications (ICONIC), 2024.
- Sanaullah, Kaushik Roy, Ulrich RĂĽckert, and Thorsten Jungeblut.” Selection of Optimal Neural Model using Spiking Neural Network for Edge Computing.” In 44th IEEE International Conference on Distributed Computing Systems (ICDCS), 2024
Competition
- Sanaullah, Hasina Attaullah, and Thorsten Jungeblut. “The Next-Gen Interactive Runtime Simulator for Neural Network Programming.” International Conference on Programming 2024.
Workshops
- Shamini Koravuna and Ulrich Rückert „Spiking Binary Associative Memory for Implementing on Neuromorphic Hardware. “In KI 2021 44th German Conference on Artificial Intelligence. Workshop on Trustworthy AI in the Wild (Sept. 27, 2021). 2021.
- Federico Pennino, Shamini Koravuna, Christoph Ostrau, and Ulrich RĂĽckert. N-mnist object recognition with spiking neural networks. In Dataninja Spring School, Poster Presentation, 2022. (Best Poster Award)
- Sanaullah, Shamini Koravuna, Ulrich RĂĽckert, and Thorsten Jungeblut. “Real-Time Resource Efficient Simulator for SNNs-based Model Experimentation.” Dataninja Spring School-Workshop 2022.
- Sanaullah, Shamini Koravuna, Ulrich RĂĽckert, and Thorsten Jungeblut. “Evaluating Spiking Neural Network Models: A Comparative Performance Analysis.” Dataninja Spring School Workshop 2023.
Tools / Simulators:
- Sanaullah et. al. “RAVSim: Run-Time Analysis and Visualization Simulator,” published by National Instruments LabVIEW.
- Sanaullah et. al. “Brain-Analysis-Simulator”, publicly available at: https://github.com/Rao-Sanaullah/Brain- Analysis-Simulator