The faces behind project ML4ProM
The Future of Business: Improving Processes and Customer Experiences with AI
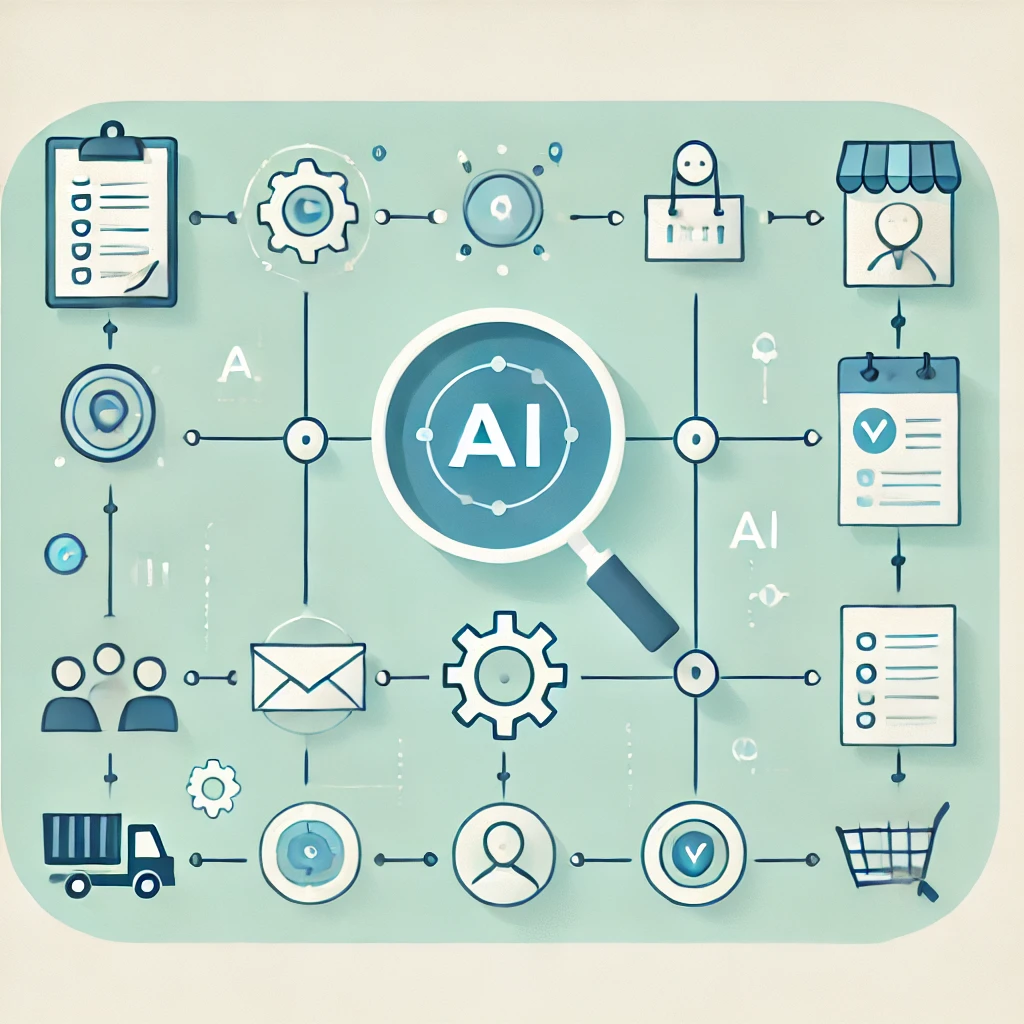
Organizations and companies store vast amounts of data about their processes and operations. Take, for example, the process of handling applications at a state insurance agency. The goal is to evaluate applicants who seek social support during difficult times, such as unemployment or disability. Our job is to dive into the recorded data from thousands of insurance cases and develop automated methods to gain insights, identify problems, and help companies improve their processes.
Typically, business processes have a planned structure, but in reality, things often don’t go exactly as planned.
Our research focuses on identifying unwanted behaviors by analyzing the available historical data. To achieve this, we use prior knowledge and common sense to highlight the differences between good and bad cases.
Additionally, we compare different cases and provide diagnoses that help experts better understand problems in their workflows. These valuable insights not only shed light on historical patterns but also serve as a guide for companies to improve their processes by learning from their own experiences.
We also use our findings from process modeling to ensure businesses run smoothly online, such as in e-commerce. For this, we’ve developed special AI-powered programs that offer product recommendations—like a friend suggesting a good book. Our programs not only use historical data but also analyze the products themselves to keep learning and improving.
What makes our work special is that we can detect when our programs make mistakes! In both areas, we focus on providing trustworthy solutions that offer clear explanations for detected process deviations and product recommendations. This gives our research a significant advantage over typical AI systems, which are often not transparent or difficult to understand.
Cooperation
Project Publications
- Artelt, André, Valerie Vaquet, Riza Velioglu, Fabian Hinder, Johannes Brinkrolf, Malte Schilling, and Barbara Hammer (2021). ‘‘Evaluating robustness of counterfactual explanations’’. In: IEEE Symposium Series on Computational Intelligence (SSCI).
- Eichenberger, Christian, Moritz Neun, Henry Martin, Pedro Herruzo, Markus Spanring, Yichao Lu, Sungbin Choi, Vsevolod Konyakhin, Nina Lukashina, Aleksei Shpilman, et al. (2022). ‘‘Traffic4cast at neurips 2021-temporal and spatial few-shot transfer learning in gridded geo-spatial processes’’. In: NeurIPS 2021 Competitions and Demonstrations Track.
- Norouzifar, Ali and Wil M. P. van der Aalst (2023). ‘‘Discovering Process Models that Support Desired Behavior and Avoid Undesired Behavior’’. In:Proceedings of the 38th ACM/SIGAPP Symposium on Applied Computing, SAC 2023, Tallinn, Estonia, March 27-31, 2023. Ed. by Jiman Hong, Maart Lanperne, Juw Won Park, Tomás Cerný, and Hossain Shahriar. ACM, pp. 365–368. doi: 10.1145/3555776.3577818. url:https://doi.org/10.1145/3555776.3577818.
- Norouzifar, Ali and Wil M. P. van der Aalst (2024). ‘‘Leveraging Desirable and Undesirable Event Logs in Process Mining Tasks’’. In: DataNinja sAIOnARA 2024 Conference abs/2408.17316, pp. 32–35. doi: 10.11576/dataninja-1164. url: https://doi.org/10.11576/dataninja-1164.
- Norouzifar, Ali, Marcus Dees, and Wil M. P. van der Aalst (2024). ‘‘Imposing Rules in Process Discovery: An Inductive Mining Approach’’. In: Research Challenges in Information Science – 18th International Conference, RCIS 2024, Guimarães, Portugal, May 14-17, 2024, Proceedings, Part I. Ed. by João Araújo, Jose Luis de la Vara, Maribel Yasmina Santos, and Said Assar. Vol. 513. Lecture Notes in Business Information Processing. Springer, pp. 220–236. doi: 10.1007/978-3-031-59465-6\_14. url: https://doi.org/10.1007/978-3-031-59465-6%5C_14.
- Norouzifar, Ali, Humam Kourani, Marcus Dees, and Wil M. P. van der Aalst (2024). ‘‘Bridging Domain Knowledge and Process Discovery Using Large Language Models’’. In: Proceedings of the 22nd International Conference on Business Process Management, in print.
- Norouzifar, Ali, Majid Rafiei, Marcus Dees, and Wil M. P. van der Aalst (2024a). ‘‘An Framework for Explainable Process Variant Analysis on Continuous Features’’. submitted.
- Norouzifar, Ali, Majid Rafiei, Marcus Dees, and Wil M. P. van der Aalst (2024b). ‘‘Process Variant Analysis Across Continuous Features: A Novel Framework’’. In: Enterprise, Business-Process and Information Systems Modeling – 25th International Conference, BPMDS 2024, and 29th International Conference, EMMSAD 2024, Limassol, Cyprus, June 3-4, 2024, Proceedings. Ed. by Han van der Aa, Dominik Bork, Rainer Schmidt, and Arnon Sturm. Vol. 511. Lecture Notes in Business Information Processing. Springer, pp. 129–142. doi: 10.1007/978-3-031-61007-3\_11. url: https://doi.org/10.1007/978-3-031-61007-3%5C_11.
- Özdemir, Özgür, Emre Salih Akın, Rıza Velioğlu, and Tuğba Dalyan (2022). ‘‘A comparative study of neural machine translation models for Turkish language’’. In: Journal of Intelligent & Fuzzy Systems.
- Velioglu, Riza, Petra Bevandic, Robin Chan, and Barbara Hammer (2024). ‘‘Clothify: Redefining Fashion E-Commerce Photography with Virtual Try-Off and Diffusion Models’’. submitted to: IEEE/CVF international conference on computer vision.
- Velioglu, Riza, Robin Chan, and Barbara Hammer (2024). ‘‘FashionFail: Addressing Failure Cases in Fashion Object Detection and Segmentation’’. In: International Joint Conference on Neural Networks (IJCNN). IEEE.
- Velioglu, Riza, Jan Philip Göpfert, André Artelt, and Barbara Hammer (2022). ‘‘Explainable Artificial Intelligence for Improved Modeling of Processes’’. In: Intelligent Data Engineering and Automated Learning (IDEAL).